How does big data help us tackle childhood cancer?
It’s our job to break down barriers, to access and to knowledge, and put big data and powerful methods in the hands of pediatric cancer experts poised for the next big discovery. Last month, the Childhood Cancer Data Lab’s (CCDL) peer-reviewed paper, MultiPLIER: A Transfer Learning Framework for Transcriptomics Reveals Systemic Features of Rare Disease, was published by Cell Systems (Taroni et al. 2019.). MultiPLIER is a machine learning approach that brings big data to bear on rare diseases. It’s also an example of the scientific approach and ethos of the CCDL, and the publication is a great opportunity to share how the CCDL is developing new technologies to accelerate research into cures for childhood cancers!
What kind of data and why did we use it?
There are many ways to measure biological samples, and these produce different types of data. In the context of MultiPLIER, “big data” refers to a type of data called transcriptomic or gene expression data. These are measurements of the collection of RNA molecules in a sample. Before we go too much further, let’s talk about why this matters.
There is something called the central dogma of molecular biology (if this gets too deep into biology jargon, fear not, the next paragraph has an example with baked goods 🧁🧁🧁), which says that DNA gives rise to RNA which gives rise to protein. Our genetic material, DNA, is made up in part by genes that encode proteins. Proteins are what do most of the work in our cells, tissues, and ultimately organs. They’re responsible for carrying out essential molecular processes like creating energy, forming structures, and signaling between different parts of our bodies. RNA is an intermediate step between DNA and proteins. A copy of RNA or a transcript (messenger RNA to be specific) is made from the DNA template when a cell needs a protein to make a structure, to transmit a signal, or to carry out a process. When this happens, we refer to this as the gene being transcribed or expressed. This is where the terms transcriptomic or gene expression arise from. We call the collection of DNA and proteins the genome and proteome, respectively. Measuring RNA gives us a snapshot of what cells are doing and is a powerful tool to gain insight into the biology of cancers. Such measurements have led to prognostic tests in adult cancers (e.g., the Prosigna Breast Cancer Prognostic Gene Signature Assay).
To use my favorite central dogma analogy (because cupcakes 🧁):

DNA is like a cookbook; it contains information about every product you could make. RNA is a like a recipe; it is how to make a particular product. Proteins are like cupcakes—the product itself. You can think of cells or tissues like a bakery, a collection of products together.
Imagine that for every individual product (cupcake) you make, you’d make a copy of the recipe. So if you were to look at all the recipes in the bakery at one point in time, you’d be able to guess what was being made. If there were many more copies of the wedding cake recipe than the cupcake recipe on the counters, you’d guess that there were lots of weddings that week.
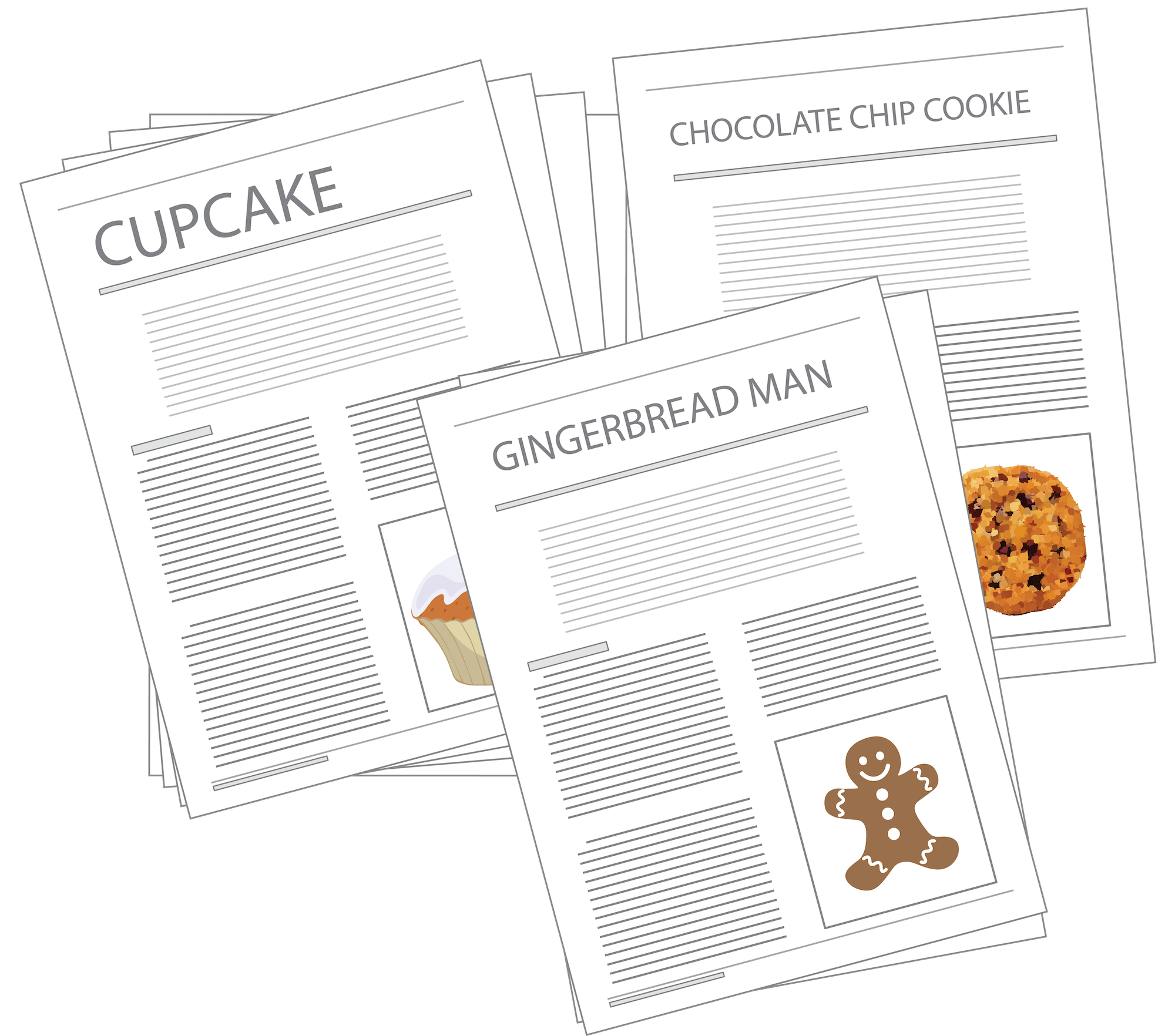
When we look at genome-wide gene expression data, we’re looking at the collection of recipes, RNA, at the time of sampling. Proteins do not usually work alone. Rather, they often work together as parts of complex pathways or processes. A baked cupcake isn’t quite ready for prime time if it doesn’t have its icing. If many of the genes that encode proteins for a particular process are expressed in a tissue (the cupcake AND the icing), we guess that this process is active in this tissue. With MultiPLIER we can extract patterns from genome-wide gene expression data (if we have a large enough sample size; more on that below). This provides a snapshot of the processes that are happening in a tissue, which could be a tumor at the time of biopsy.
MultiPLIER is a more powerful method to study rare diseases
When studying childhood cancers, we’ve faced two major challenges that MultiPLIER addresses:
- Individual childhood cancer diagnoses are rare diseases. This limits the sample size for an individual study. It is difficult to find important patterns in small datasets.
- Multiple datasets = multiple models. For example, we often compare results across groups of patients from different institutions because results that show up repeatedly are more likely to be true. This is time consuming and limits the number of studies that we can look at.
We show in the peer-reviewed paper that the MultiPLIER strategy learns many more pathways than analysis of individual datasets. This means that it provides a higher resolution view into what's happening in a disease. Also, because we learn a single model, which we can then apply across datasets, we no longer have to do that time-consuming comparison step.
Brief Aside - What is a MultiPLIER model? The standard approach to using tools like PLIER is to extract patterns from single datasets. For MultiPLIER, we train a machine learning model using a method called PLIER (Mao et al. bioRxiv. 2017.) on as many datasets as we have available to us (Multi-dataset PLIER, or MultiPLIER for short), even if the datasets come from completely different diseases than what we want to study. The output of PLIER is patterns of genes that tend to vary together in their expression levels. When we trained the MultiPLIER model, a couple of things might have happened. We might have found that training over different datasets muddled all of the signals and so we got less resolution than with individual datasets. Alternatively, we might have found that we got high resolution, but not for the pathways and processes relevant for the diseases we wanted to study. Fortunately, what we ended up with was a model that provided higher resolution than PLIER provided for analyses within a single dataset or disease and also one that described the features, like a neutrophil signature, of rare diseases—even when those rare diseases weren’t provided to the model during training.
Validation, simplified
In the past when we’ve studied pathways across datasets, we had to perform an analysis within each dataset and then compare across them. MultiPLIER has many uses, but one that we’re excited about is that it provides a single model that can be applied across many datasets. Going back to the bakery analogy, it’s like we can now show up at any bakery and while just glancing through the window, we can get a quick summary of the relative quantities of cupcakes, cookies, and wedding cakes. The MultiPLIER model smooths out differences in the individual shops and lets us focus on the important differences.
For example, we were able to identify concordance between two medulloblastoma cohorts, Robinson et al. and Northcott et al., with relative ease—check out the figure below. (As a side note for those who know a bit about gene expression platforms, the MultiPLIER model was trained entirely on RNA-seq data, and the medulloblastoma datasets are measured on the older microarray technology; the approach is able to overcome the cross-technology noise). We could quickly study the role of pathways across medulloblastoma subtypes in two different datasets.
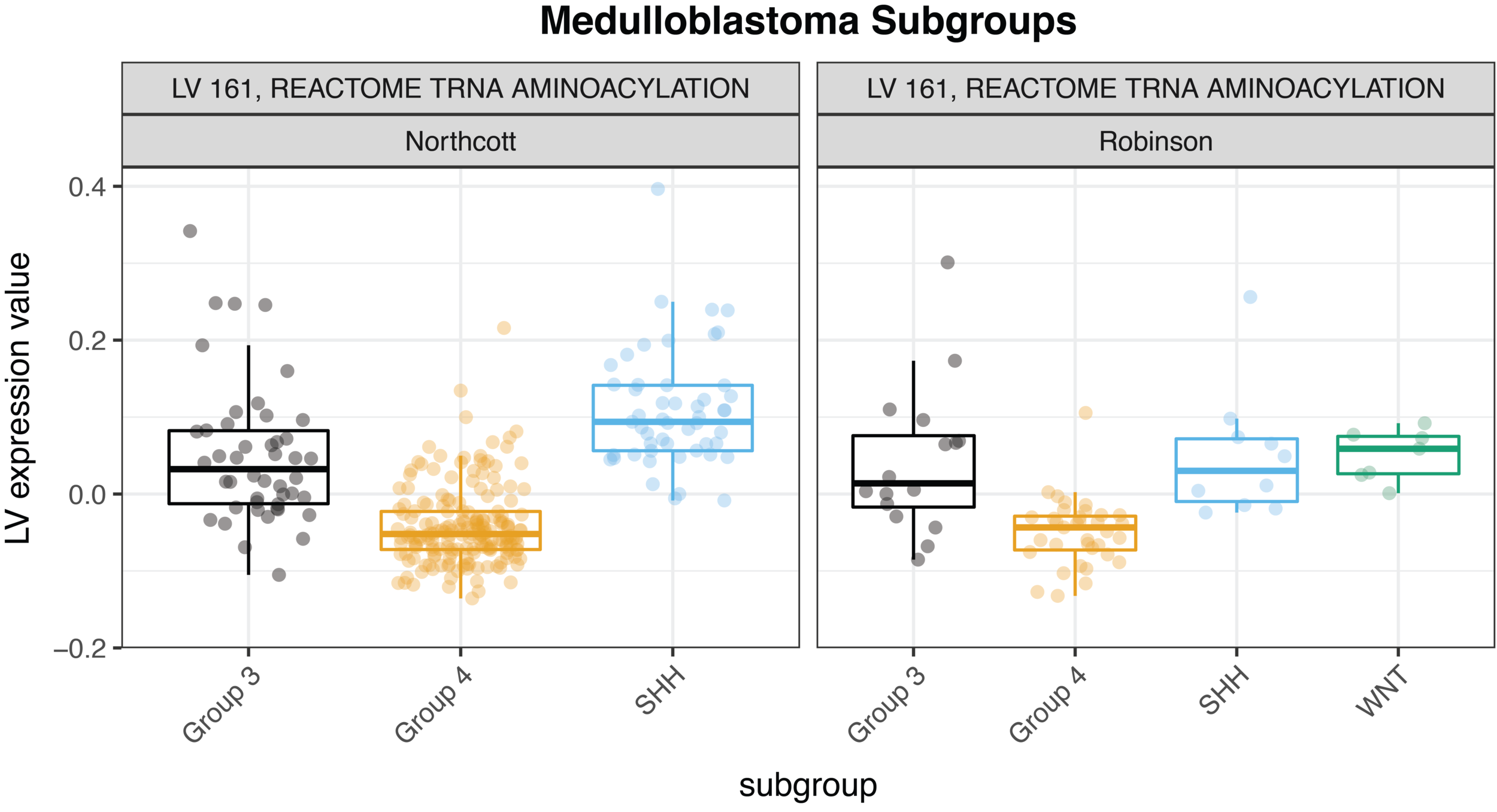
Our comparisons are not limited to different cohorts examining the same disease. We could also study these patterns across multiple pediatric cancers, which is a direction we are now exploring. MultiPLIER is a tool that makes it much faster to do the robust work of multi-dataset comparisons that are so essential to finding new targets or vulnerabilities of rare cancers!
MultiPLIER and the CCDL ethos
Some of the parts that make me most proud of the work we did with the MultiPLIER project are things that we don’t always talk about as members of the scientific community. However, these same parts are intrinsic to the spirit of the CCDL.
It’s important to us at the CCDL that we’re bringing tools and conceptual advances to the study of pediatric cancers. The MultiPLIER project would have been impossible, full stop, without the work of other scientists. In particular, we owe a great deal of gratitude to the scientists who shared their gene expression data publicly, researchers who built recount2 (Collado-Torres et al. Nature Biotechnology. 2017.), which we used as training data for the MultiPLIER model, and the authors of PLIER (Mao et al. bioRxiv. 2017.).
We made all of our code and models from this project open, easy to access, and easy to build upon via permissive licensing. You can take a look at all the code here: https://github.com/greenelab/multi-plier. You can even see our negative results—the things that didn’t work. This helps other researchers understand when this approach may fail for them and can save them time they would have invested in the research equivalent of a dead-end. We know folks are already using this approach for their research because we’ve communicated with and helped them along the way.
What’s next?
The resources underlying MultiPLIER, recount2 and PLIER, were set up for studying human data (at least at the inception of the project). We know that if we are to discover new treatments for childhood cancers, we’ll also need to draw on studies in model organisms because these systems are powerful tools for probing biological processes or screening compounds. We’ve been building refine.bio with childhood cancers in mind: we’re processing gene expression samples from model organisms such as zebrafish and mouse, and because these diseases are rare we’re processing both microarray and RNA-seq samples to unlock as much data as possible. We’ve also developed strategies to make it easier to use PLIER with model organisms (see this notebook). We’re looking forward to trying the MultiPLIER approach in zebrafish to study models of pediatric cancer, and we’re excited to see where other researchers take this next.
It’s our job to break down barriers, to access and to knowledge, and put big data and powerful methods in the hands of pediatric cancer experts poised for the next big discovery. Last month, the Childhood Cancer Data Lab’s (CCDL) peer-reviewed paper, MultiPLIER: A Transfer Learning Framework for Transcriptomics Reveals Systemic Features of Rare Disease, was published by Cell Systems (Taroni et al. 2019.). MultiPLIER is a machine learning approach that brings big data to bear on rare diseases. It’s also an example of the scientific approach and ethos of the CCDL, and the publication is a great opportunity to share how the CCDL is developing new technologies to accelerate research into cures for childhood cancers!
What kind of data and why did we use it?
There are many ways to measure biological samples, and these produce different types of data. In the context of MultiPLIER, “big data” refers to a type of data called transcriptomic or gene expression data. These are measurements of the collection of RNA molecules in a sample. Before we go too much further, let’s talk about why this matters.
There is something called the central dogma of molecular biology (if this gets too deep into biology jargon, fear not, the next paragraph has an example with baked goods 🧁🧁🧁), which says that DNA gives rise to RNA which gives rise to protein. Our genetic material, DNA, is made up in part by genes that encode proteins. Proteins are what do most of the work in our cells, tissues, and ultimately organs. They’re responsible for carrying out essential molecular processes like creating energy, forming structures, and signaling between different parts of our bodies. RNA is an intermediate step between DNA and proteins. A copy of RNA or a transcript (messenger RNA to be specific) is made from the DNA template when a cell needs a protein to make a structure, to transmit a signal, or to carry out a process. When this happens, we refer to this as the gene being transcribed or expressed. This is where the terms transcriptomic or gene expression arise from. We call the collection of DNA and proteins the genome and proteome, respectively. Measuring RNA gives us a snapshot of what cells are doing and is a powerful tool to gain insight into the biology of cancers. Such measurements have led to prognostic tests in adult cancers (e.g., the Prosigna Breast Cancer Prognostic Gene Signature Assay).
To use my favorite central dogma analogy (because cupcakes 🧁):

DNA is like a cookbook; it contains information about every product you could make. RNA is a like a recipe; it is how to make a particular product. Proteins are like cupcakes—the product itself. You can think of cells or tissues like a bakery, a collection of products together.
Imagine that for every individual product (cupcake) you make, you’d make a copy of the recipe. So if you were to look at all the recipes in the bakery at one point in time, you’d be able to guess what was being made. If there were many more copies of the wedding cake recipe than the cupcake recipe on the counters, you’d guess that there were lots of weddings that week.
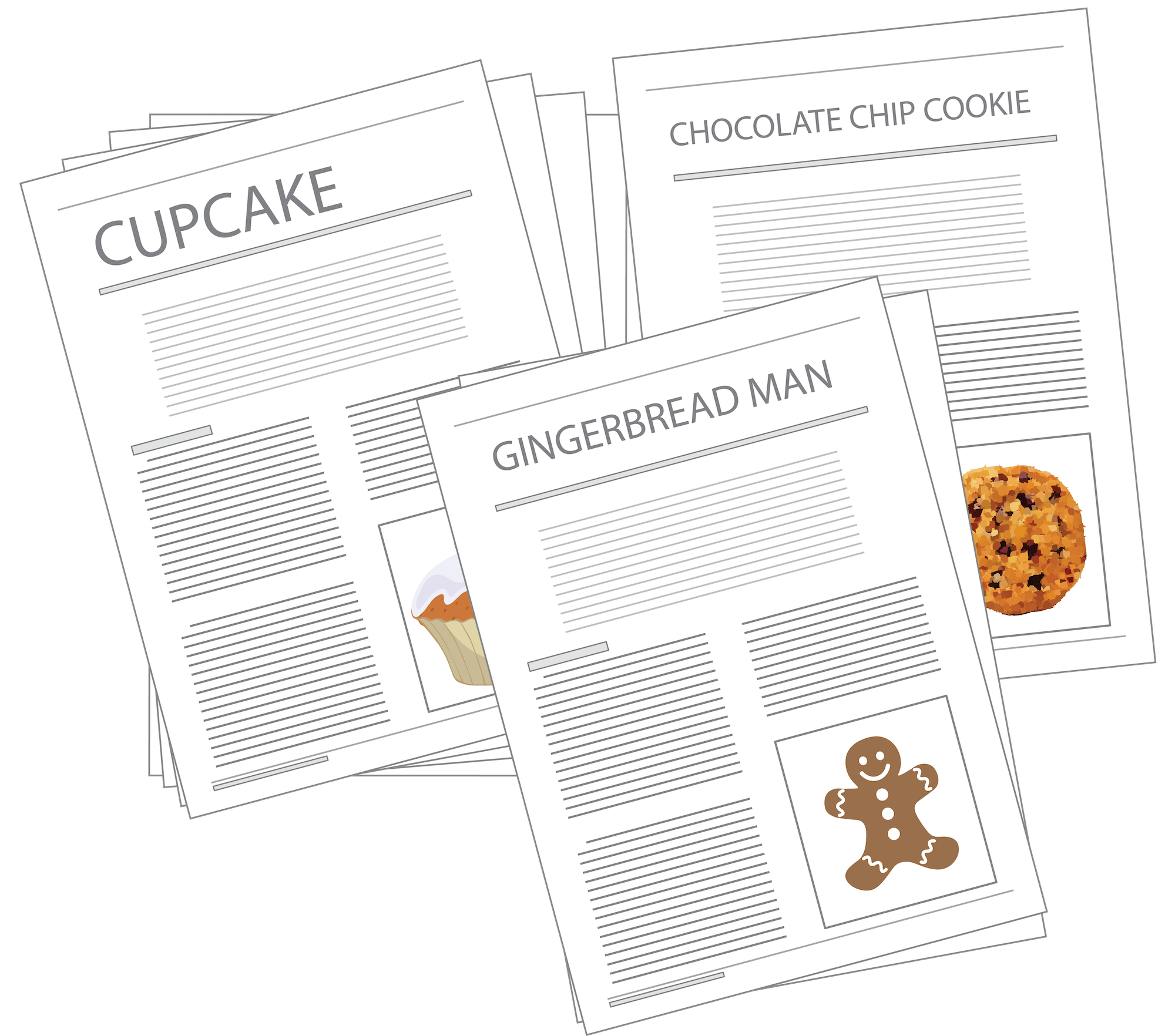
When we look at genome-wide gene expression data, we’re looking at the collection of recipes, RNA, at the time of sampling. Proteins do not usually work alone. Rather, they often work together as parts of complex pathways or processes. A baked cupcake isn’t quite ready for prime time if it doesn’t have its icing. If many of the genes that encode proteins for a particular process are expressed in a tissue (the cupcake AND the icing), we guess that this process is active in this tissue. With MultiPLIER we can extract patterns from genome-wide gene expression data (if we have a large enough sample size; more on that below). This provides a snapshot of the processes that are happening in a tissue, which could be a tumor at the time of biopsy.
MultiPLIER is a more powerful method to study rare diseases
When studying childhood cancers, we’ve faced two major challenges that MultiPLIER addresses:
- Individual childhood cancer diagnoses are rare diseases. This limits the sample size for an individual study. It is difficult to find important patterns in small datasets.
- Multiple datasets = multiple models. For example, we often compare results across groups of patients from different institutions because results that show up repeatedly are more likely to be true. This is time consuming and limits the number of studies that we can look at.
We show in the peer-reviewed paper that the MultiPLIER strategy learns many more pathways than analysis of individual datasets. This means that it provides a higher resolution view into what's happening in a disease. Also, because we learn a single model, which we can then apply across datasets, we no longer have to do that time-consuming comparison step.
Brief Aside - What is a MultiPLIER model? The standard approach to using tools like PLIER is to extract patterns from single datasets. For MultiPLIER, we train a machine learning model using a method called PLIER (Mao et al. bioRxiv. 2017.) on as many datasets as we have available to us (Multi-dataset PLIER, or MultiPLIER for short), even if the datasets come from completely different diseases than what we want to study. The output of PLIER is patterns of genes that tend to vary together in their expression levels. When we trained the MultiPLIER model, a couple of things might have happened. We might have found that training over different datasets muddled all of the signals and so we got less resolution than with individual datasets. Alternatively, we might have found that we got high resolution, but not for the pathways and processes relevant for the diseases we wanted to study. Fortunately, what we ended up with was a model that provided higher resolution than PLIER provided for analyses within a single dataset or disease and also one that described the features, like a neutrophil signature, of rare diseases—even when those rare diseases weren’t provided to the model during training.
Validation, simplified
In the past when we’ve studied pathways across datasets, we had to perform an analysis within each dataset and then compare across them. MultiPLIER has many uses, but one that we’re excited about is that it provides a single model that can be applied across many datasets. Going back to the bakery analogy, it’s like we can now show up at any bakery and while just glancing through the window, we can get a quick summary of the relative quantities of cupcakes, cookies, and wedding cakes. The MultiPLIER model smooths out differences in the individual shops and lets us focus on the important differences.
For example, we were able to identify concordance between two medulloblastoma cohorts, Robinson et al. and Northcott et al., with relative ease—check out the figure below. (As a side note for those who know a bit about gene expression platforms, the MultiPLIER model was trained entirely on RNA-seq data, and the medulloblastoma datasets are measured on the older microarray technology; the approach is able to overcome the cross-technology noise). We could quickly study the role of pathways across medulloblastoma subtypes in two different datasets.
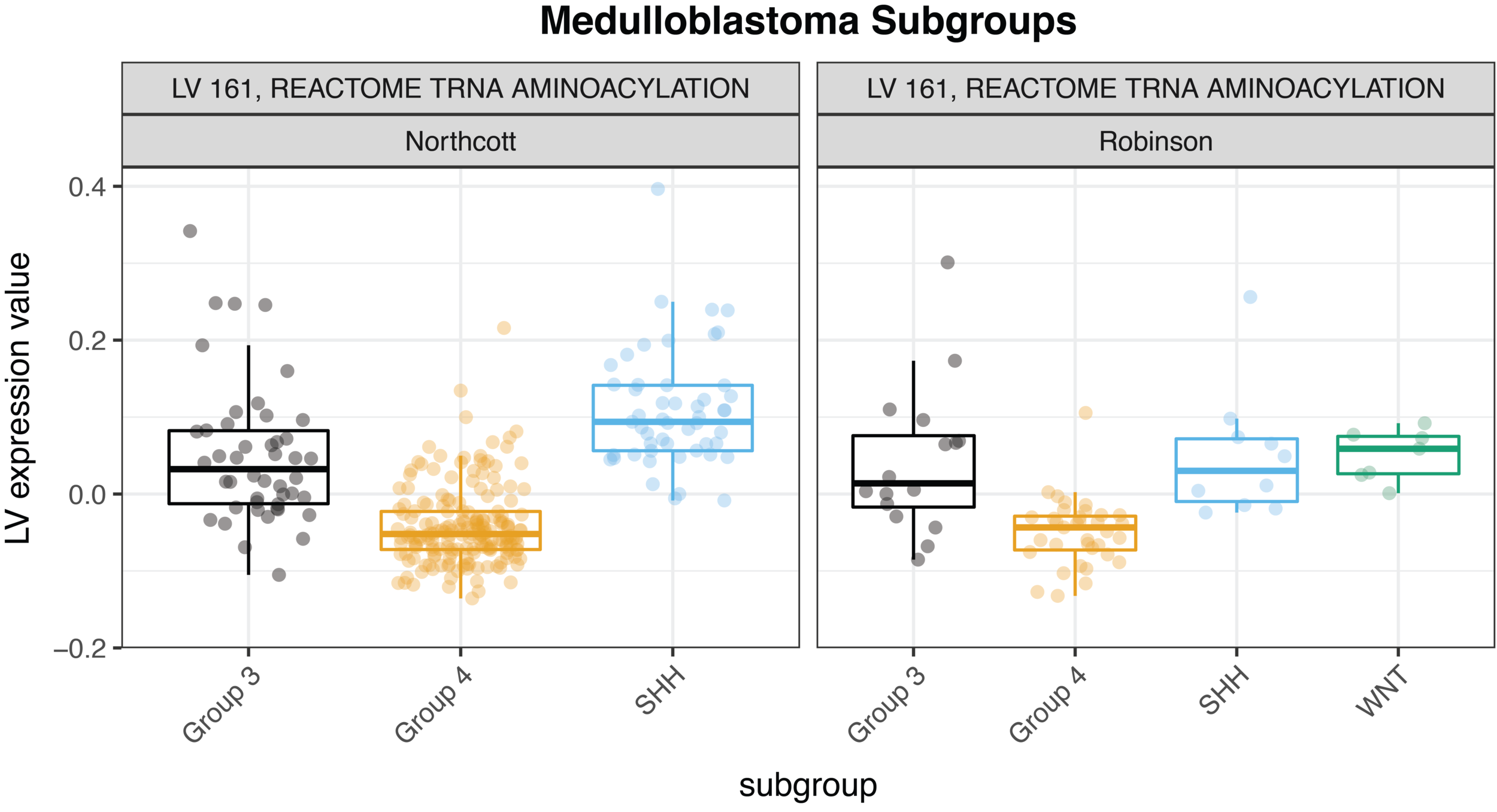
Our comparisons are not limited to different cohorts examining the same disease. We could also study these patterns across multiple pediatric cancers, which is a direction we are now exploring. MultiPLIER is a tool that makes it much faster to do the robust work of multi-dataset comparisons that are so essential to finding new targets or vulnerabilities of rare cancers!
MultiPLIER and the CCDL ethos
Some of the parts that make me most proud of the work we did with the MultiPLIER project are things that we don’t always talk about as members of the scientific community. However, these same parts are intrinsic to the spirit of the CCDL.
It’s important to us at the CCDL that we’re bringing tools and conceptual advances to the study of pediatric cancers. The MultiPLIER project would have been impossible, full stop, without the work of other scientists. In particular, we owe a great deal of gratitude to the scientists who shared their gene expression data publicly, researchers who built recount2 (Collado-Torres et al. Nature Biotechnology. 2017.), which we used as training data for the MultiPLIER model, and the authors of PLIER (Mao et al. bioRxiv. 2017.).
We made all of our code and models from this project open, easy to access, and easy to build upon via permissive licensing. You can take a look at all the code here: https://github.com/greenelab/multi-plier. You can even see our negative results—the things that didn’t work. This helps other researchers understand when this approach may fail for them and can save them time they would have invested in the research equivalent of a dead-end. We know folks are already using this approach for their research because we’ve communicated with and helped them along the way.
What’s next?
The resources underlying MultiPLIER, recount2 and PLIER, were set up for studying human data (at least at the inception of the project). We know that if we are to discover new treatments for childhood cancers, we’ll also need to draw on studies in model organisms because these systems are powerful tools for probing biological processes or screening compounds. We’ve been building refine.bio with childhood cancers in mind: we’re processing gene expression samples from model organisms such as zebrafish and mouse, and because these diseases are rare we’re processing both microarray and RNA-seq samples to unlock as much data as possible. We’ve also developed strategies to make it easier to use PLIER with model organisms (see this notebook). We’re looking forward to trying the MultiPLIER approach in zebrafish to study models of pediatric cancer, and we’re excited to see where other researchers take this next.