Accelerating the Pace of Childhood Cancer Research with Big Data
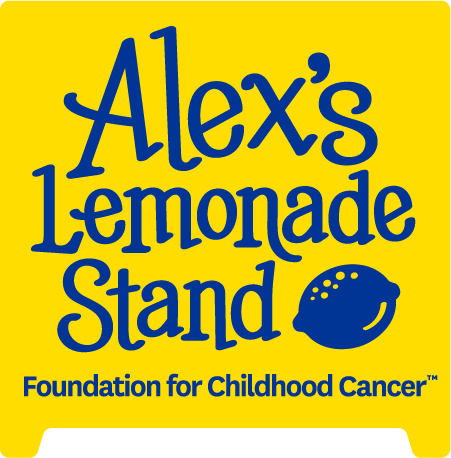
The Childhood Cancer Data Lab was established by Alex’s Lemonade Stand Foundation (ALSF) in 2017. ALSF recognized that pediatric cancer researchers face hurdles that impede the pace of research.
ALSF introduced the Data Lab to empower researchers and scientists across the globe by removing roadblocks, supporting opportunities for collaboration and sharing, and developing resources to accelerate new treatment and cure discovery.
The Data Lab's mission is to empower pediatric cancer experts poised for the next big discovery with the knowledge, data, and tools to reach it. We construct tools that make vast amounts of data widely available, easily mineable, and broadly reusable. We train researchers and scientists to better understand their own data and to advance their work more quickly.
To date, the Data Lab has trained over 200 childhood cancer researchers and has harmonized over 1.3 million data samples and made them easily available. Learn more about the Data Lab’s impact here.
Projects
The Data Lab develops tools designed to make data and analysis widely available and broadly reusable.
Data Science Workshops
The Data Lab offers workshops to teach researchers the data science skills they need to examine their own data. Our courses focus on the most cutting edge tools and analysis techniques. We ensure that participants walk away with an understanding of:
- The R programming language, R Notebooks, and some reproducible research practices.
- Processing bulk and single-cell RNA-seq data from raw all the way to downstream analyses.
- Downstream analyses methods like differential expression analyses, hierarchical clustering, and preparing publication-ready plots.
“I think anyone who is working on or near single-cell data should take this course. I am so much more confident in what I understand about single-cell analyses compared to where I was at the beginning. 10/10 recommend.”
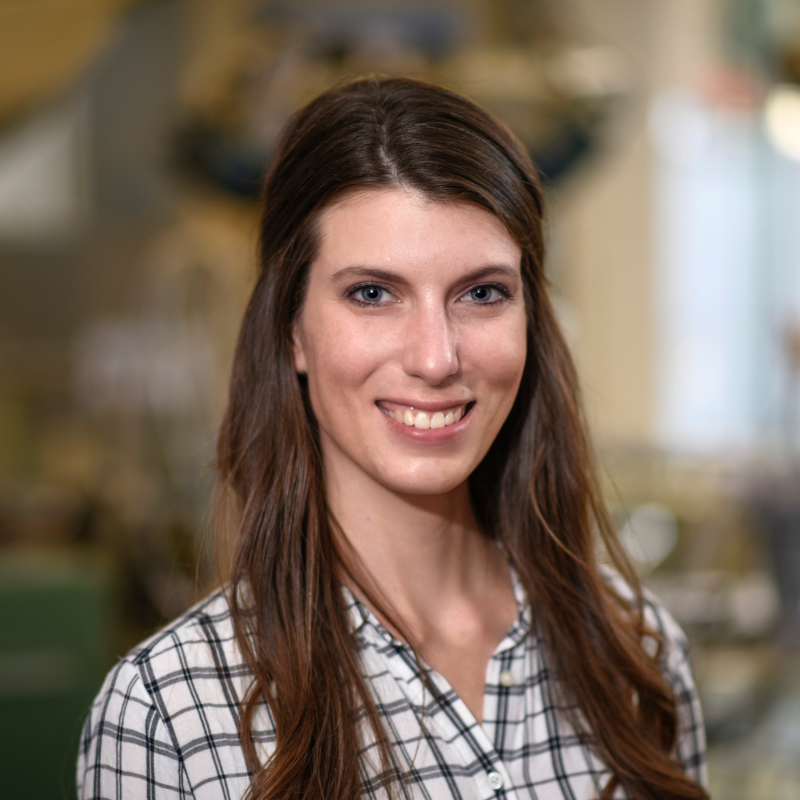
Donate
Make a donation to support the Data Lab’s mission of putting knowledge and resources in the hands of pediatric cancer experts poised for the next big discovery.
With your help, we can
Fund innovative models to scale training workshops.
Offer our expertise and provide consultation on projects that will change the future for children fighting cancer.
Train at least 200 childhood cancer researchers over the next four years.
Blog
The Data Lab has just launched the brand new Open Single-cell Pediatric Cancer Atlas (OpenScPCA) project! This open, collaborative project aims to analyze data from the ScPCA Portal, which currently holds 500 samples from over 50 pediatric cancer types. We are seeking contributors with diverse skills and expertise to join the project!
We are excited to announce that our next virtual workshop, Introduction to Single-cell RNA-Seq, will run from June 10-14, 2024! In this workshop, Data Lab staff will introduce researchers studying pediatric cancer to the R programming language, the Tidyverse R packages for data science, single-cell RNA-seq data analysis, and annotating cell types.
Applications are open for the Data Lab's next workshop! We are holding a two-day course on Reproducible Research Practices and the Open Single-cell Pediatric Cancer Atlas (OpenScPCA) project from May 14-15, 2024. Please note that the OpenScPCA module is an optional part of the workshop. The course begins with an introduction to principles and techniques to achieve reproducible results in computational cancer research. On day two, you can choose to continue the workshop and learn how to put your skills to use for OpenScPCA, our new pediatric cancer research project.